Does Data Science Have a Future?
- ritulagad123
- Jan 9, 2023
- 2 min read
Data Science future
Many in the business find it difficult to understand how something as lucrative-sounding as data science can ever be regarded as dead given that it has been called "the most promising vocation'' by LinkedIn and the "best job in America" by Glassdoor. According to its description, "Data science is a discipline of study that combines domain knowledge, programming abilities, and knowledge of mathematics and statistics to extract useful insights from data."
Therefore, data science as a field won't go out of style any time soon—at least not until we figure out a method to stop using data altogether. Many, however, think that because a data scientist's everyday responsibilities are quantitative or statistical, they may be automated and that a data scientist will not be required in the future.
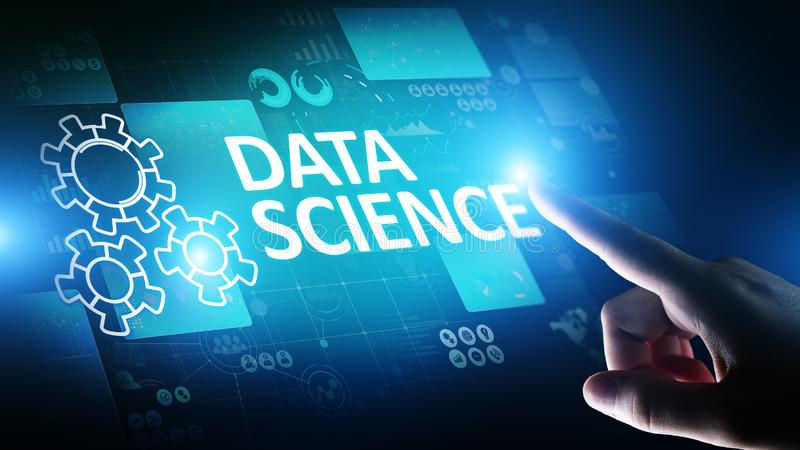
Domain knowledge is important
The idea was inspired by the fact that auto ml models may partially automate some data scientist jobs, including model construction, visualization of data, and data purification. Although the technologies may be capable of performing the task effectively, many do not pay attention to the requirement for "domain expertise" in the definition of a data scientist.
Domain expertise is the in-depth understanding of a specific topic that data scientists use to enhance their data science abilities. Therefore, even when a sizable portion of the data pipeline and workflow is automated, a data scientist is still required to convert the business problem being handled into the appropriate format.
Additionally, deciding which data science model to use based on the industry is not simple. A recommendation algorithm for the health industry would not be useful for a video streaming platform, in particular given the stark differences between the two industries.
Applying the proper context to a model, according to Tina, a former data scientist at Meta, is arguably the most underappreciated aspect of a data scientist's work. She described her time spent working on Instagram's integrity at Meta, saying that there was a machine learning module that checked for content integrity and demonetized users who violated the rules. In addition to obtaining the data from the model, my task was to decide what qualifies as a rule violation.
"The problem is that ML models can't detect 'unknown unknowns,' and if you can't even measure anything, how can you tell whether it's even breaking the law," says Tina. There is always a delicate balance between honesty and free expression.
The worry first appeared in the accounting industry a few years ago when it was suggested that AI would replace the work of accountants and auditors. Even if an AI program can essentially perform all accounting tasks, you still need an accountant's knowledge of tax breaks, credits, and other benefits.
Similarly to this, a data scientist may rely on the autoML models to gather, visualize, and clean data so they can focus more on business requirements. Additionally, as data science is still in its infancy in many traditional fields including banking, healthcare, defense, and government, the need for data scientists will only rise in the future.
The amusing thing is that data must first be present for AutoML to even begin data exploration.
Comments